Guillaume Mercère
University of Poitiers, France
Revisiting gray box model learning and Kalman filtering with subspace based model identification
Streaming link:
https://eu.bbcollab.com/guest/6c0b700cbe744161975e8cce8ed0c9cb
11 June, 5pm
KU Leuven, Thermotechnisch Instituut
Aula van de Tweede Hoofdwet (01.02)
Abstract
In numerous industrial projects, engineers face the crucial task of extracting physical parameters and signals from real-world data. When employing gray box state space model learning, a key challenge lies in generating reliable initial estimates to ensure convergence towards precise parameter values. Similarly, in utilizing Kalman filtering for signal reconstruction, a common obstacle is selecting reliable covariance matrices to attain accurate estimates. This presentation delves into how subspace-based model identification can effectively address these challenges. These solutions, relying solely on linear algebra, center on determining the similarity transformation between various state space realizations. Through both simulated and real data demonstrations, we illustrate the efficacy of these approaches.
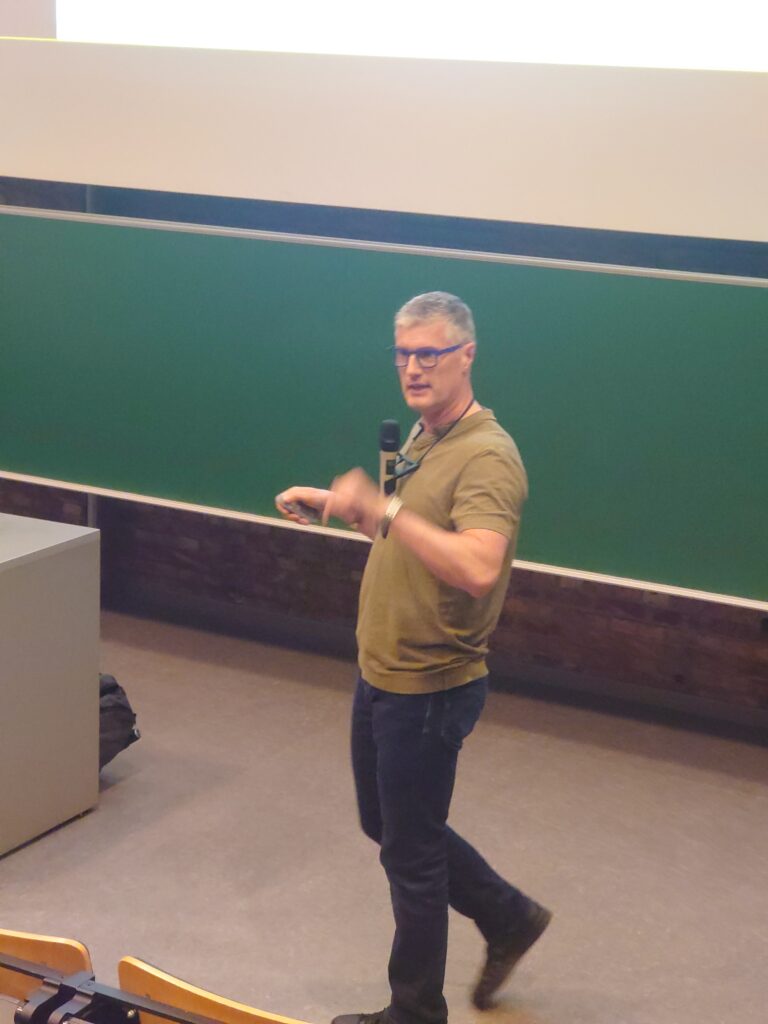
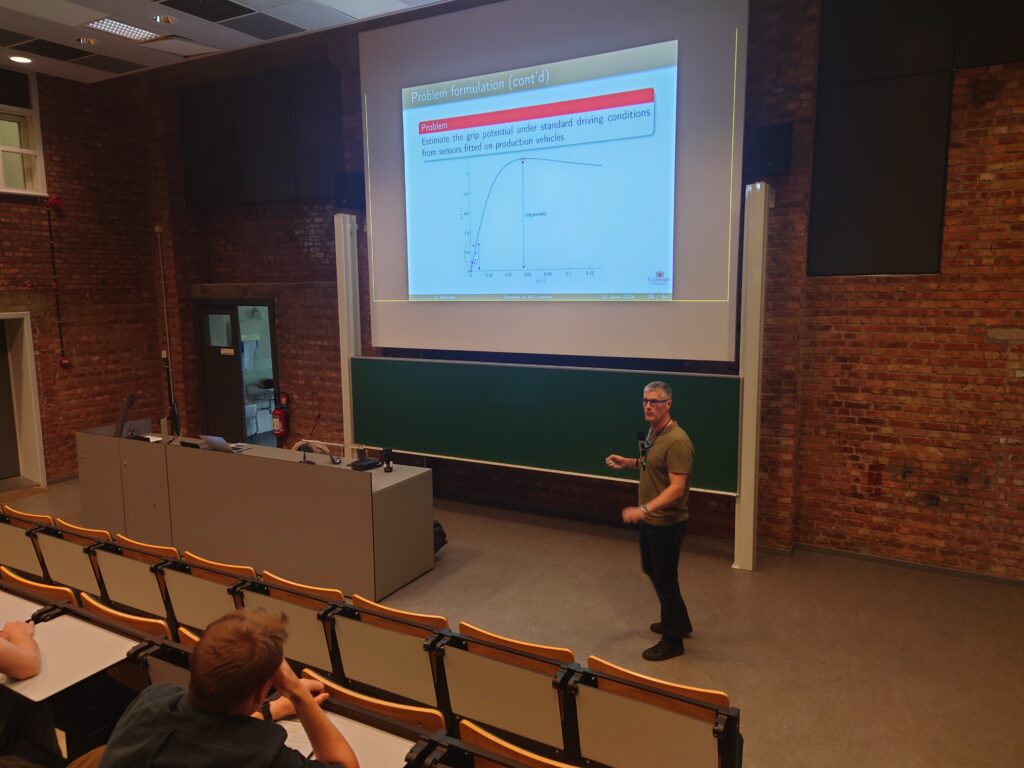
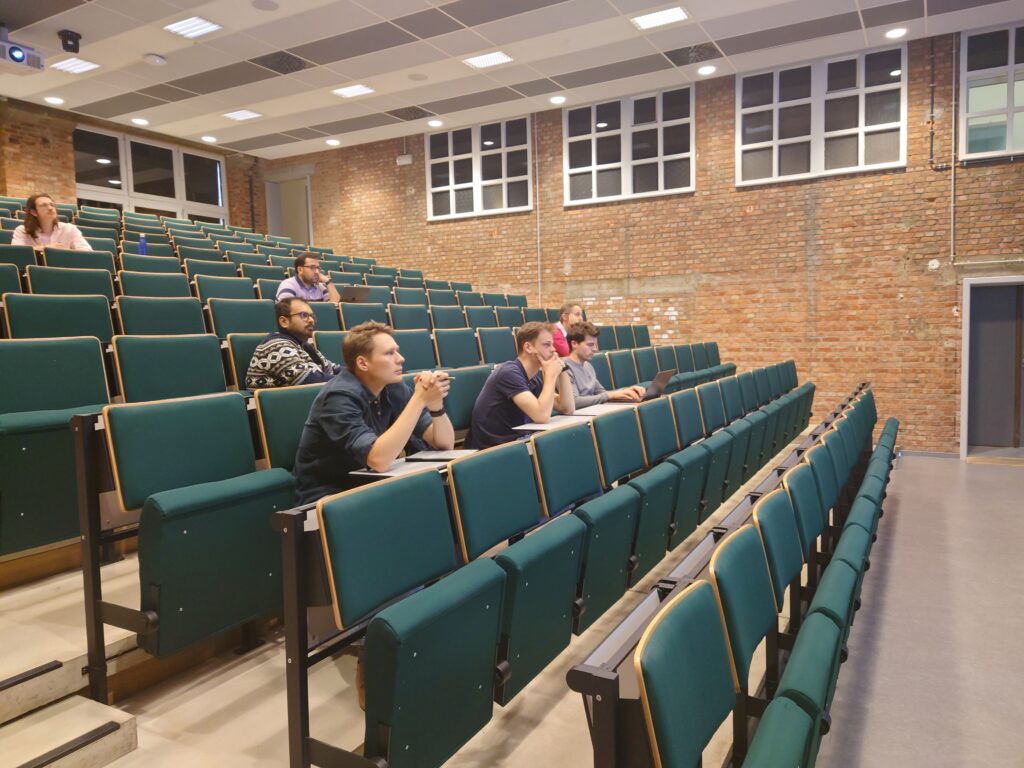
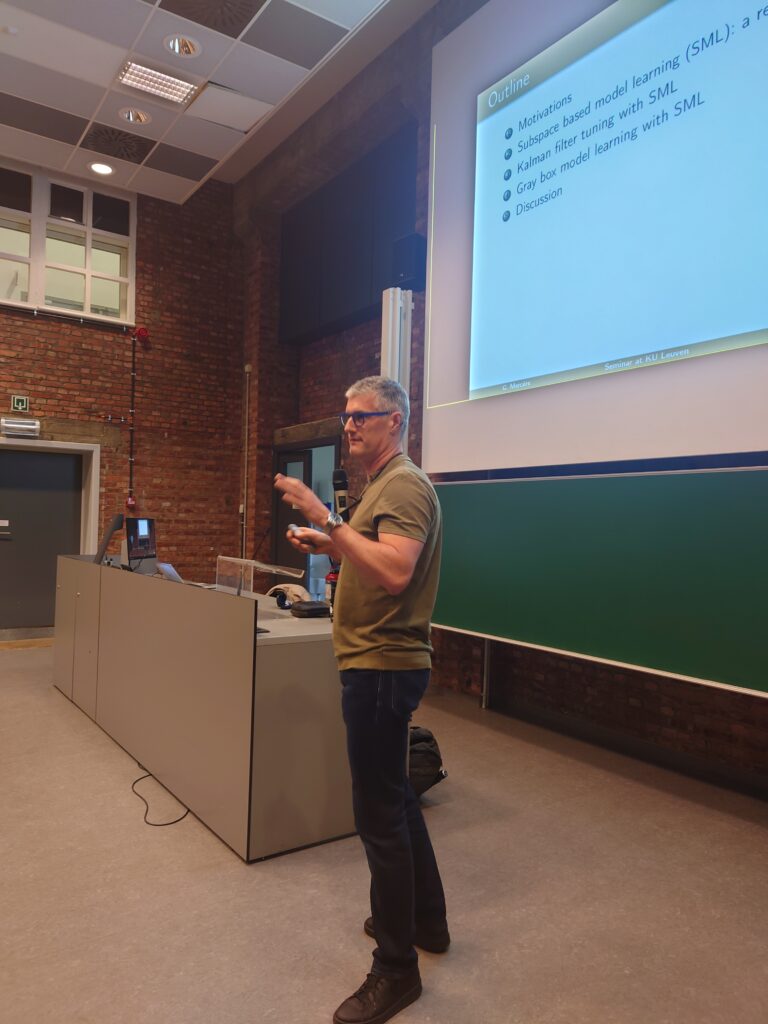